Methodologies To Drive AI In An Enterprise
Lean And Digital Thinking
Add bookmark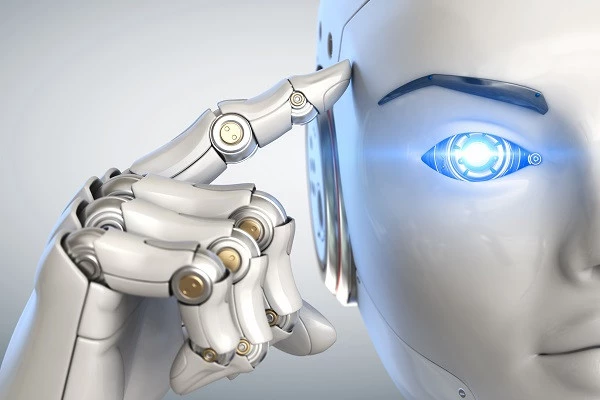
Some of the techniques that can be utilized to drive artificial intelligence (AI) innovation are lean and design thinking. Both techniques, or a modified combination, are useful to effectively innovate. In this article, I will provide an overview to both to enable you to be a practitioner, especially to employ design thinking without any tools whatsoever.
What Is Lean?
Lean is the elimination of waste, where “waste” refers to work that adds no value or limited value to a process. Lean is about organizing work activities. In the context of AI, minimizing time, improving agility, minimizing costs and rework are all benefits of lean. Some direct methodologies and outputs of Lean are cutting down redundant processes, automation and Agile/Scrum. Using lean methodologies can effectively disrupt the enterprise through predictive analytics, data mining, AI/ML technologies. Utilizing and moving big data analytics to the front further hastens decision making. These digital technologies provide significant improvements and efficiencies to the business, and can be considered extensions of lean informally. Innovation through these disruptive technologies can be quickened by utilizing the methodologies described below.
What Is Design Thinking?
Design thinking is tremendously useful in order to drive innovation. It has been successfully used in the context of new product development ideas. Design thinking is about utilizing the power of, “How might we” to challenge the status quo. Ideo is a type of design thinking methodology that goes through the steps of framing a question, gathering inspiration and ideas, making them tangible, followed by test to learn and share. A slightly modified version of ideo will provide the following stage breakdown of this methodology into:
- empathize
- define
- ideate
- prototype
- test
Challenging the status quo is as simple as asking questions and works magic for innovation, which happens anywhere and can be fostered via the right approach in a team setting of any size. The creativity of the design thinking approach is fascinating and very effective when utilized to bring forth innovation.
Execution Of A Lean AI Model
Design thinking can be used to conduct workshops. Incorporating the principles of lean and design thinking results in a model of AI that called the lean AI model. An enterprise should consider adopting this as things start moving past the initial laboratory environment.
The different steps in the execution of a lean AI model are:
- Understand: Develop a good understanding of the business problem you are trying to solve
- Engineer: Utilize appropriate agile methodologies and read data, cleanse/prepare data as well technically design your application
- Model: Apply appropriate machine learning models and evaluate accuracies
- User Feedback: This is key to ensure that the model is performing satisfactorily to meet the user’s needs, and it may be necessary to go back to the “understand” step to refine understanding
- Deploy: Once your model is optimized, you will move it to deploying the solution in a production environment
- Validate: After deployment, continuous validation is required. As newer data becomes available, it should be incorporated into the learning.
Some Illustrations In Digital Health
Prototypes utilizing the methodologies of lean and design thinking have resulted in many benefits, including finding the right solution quicker and speeding up cycle time from ideation to deployment. Some business illustrations of these techniques in a bio-pharmaceutical organization can result in key benefits, such as enabling faster patient recruitment via chatbot, utilizing real world evidence/real world data on cancer to get additional insights, predictive analytics for revenues as early indicator to speed up corrective action, and clinical trial automation to speed up tables list figures (TLF) generation from data sets. Having the innovation team re-imagine engagement with patients and doctors can result in several other use cases. For example, Twitter sentiment analysis about products, garnering real world evidence data from social media to evaluate, and social media network analysis to determine communities/cliques with respect to health care organizations for drugs/indications.
Ken Kumar is a dynamic and accomplished technology executive with significant experience and success in Life Sciences with full IT accountability to enhance shareholder and partner value. Ken is a business-driven technology leader with vision, thought leadership and management skills in analytics, artificial intelligence(AI)/machine learning, enterprise systems, and green-field implementations. Ken champions diverse projects with expertise in leadership by innovation, application delivery, architecture, system integrations, infrastructure and service desk. He is an acclaimed thought leader in digital disruption who implements technology solutions, and deliveries in AI and machine learning utilizing big data for analytics. He can be reached at ken.kaks99@gmail.com.