There is No Hype: AI Is Gaining Momentum In The Enterprise
Add bookmark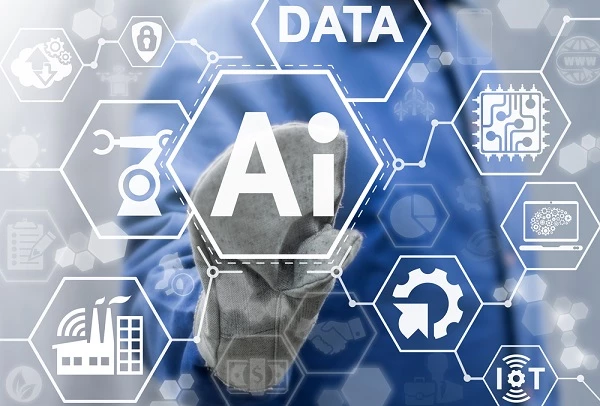
By now we’ve heard much about the value of harnessing and utilizing big data, and with the combined effect of improved algorithms and technologies like cloud and sophisticated analytics, AI is starting to make a splash in the enterprise.
IDC is projecting global spending on cognitive and AI offerings to reach $46 billion in revenues by 2020, while Statista is forecasting that the global AI market will hit $59.75 billion by 2025. Meanwhile, Accenture maintains that AI will also have a significant impact on an area not often discussed: the economy. Not only can AI make workers more efficient, but it is taking over automated tasks in the form of virtual assistants and other intelligent machines and has the potential to move the economy forward, Accenture says.
“Unlike traditional automation solutions, artificial intelligence-powered innovation automates complex physical tasks that require adaptability and agility and AI is capable of self-learning,’’ according to the Accenture report Artificial Intelligence is the Future of Growth.
Depending on who you talk to, you will likely hear differing definitions of AI. At its most basic, AI is defined as intelligent machines using technologies that can reason, interact and learn. Altimeter Group fleshes that out more; defining AI as “a set of technologies that enable machines to reproduce certain types of human capabilities; for example, the ability to see, listen, speak, move, reason, decide, predict, act, and — most importantly — learn from past experience.”
See Related: The Future Of AI And Intelligent Enterprise: Report
In an enterprise context, there are three areas where AI applications are maturing and providing value: intelligence (the ability to classify, predict, analyze, recommend, act and learn); computer vision (seeing and in tandem with intelligence, be used to interpret and extract insights from images); and conversation (AI that can listen, understand and communicate using real language), according to the newly released Altimeter report AI in the Enterprise: Real Strategies for Artificial Intelligence.
And virtually any industry can reap benefits from AI. In more than two-thirds of use cases examined by McKinsey, AI was found to enhance performance over other analytics techniques. “On average, our use cases suggest that modern deep learning AI techniques have the potential to provide a boost in additional value above and beyond traditional analytics techniques ranging from 30 percent to 128 percent, depending on industry,’’ the report Notes from the AI Frontier: Applications and Value of Deep Learning finds.
Use cases for enterprise intelligence are in customer experiences and service; sales and marketing/e-commerce; advertising, employee productivity; recruitment, IT and operations, manufacturing and supply chain, says Susan Etlinger, the report’s author. For example, online payment platform Stripe is using machine learning to detect fraud in online payments as well as for analytics and revenue forecasting.
See Related: CIOs Talk AI, Digital Pressures and Other Pain Points at MIT’s CIO Symposium
Computer vision, such as facial recognition and photo tagging, can be blended with enterprise intelligence to develop predictive models to forecast and act on emerging trends. Use cases here include brand management, such as managing quality issues like a picture of a damaged product; customer experience – providing accessibility for the visually impaired as well as the ability to do a visual search and security and surveillance, the report notes.
However, “Visual AI is not without its challenges,’’ says Etlinger. “Like other AI applications, it requires massive amounts of specific training data to enable it to accurately recognize objects, attributes, and, importantly, context. It can misinterpret images in comic, awkward, or offensive ways, as has been amply documented.”
The most widely recognized forms of conversational AI are voice agents like Siri and Alexa, and chatbots found in Skype and Messenger. “What makes conversational AI much more than a novelty, however, is that our interaction with technology is evolving — from browser-based interactions on computers or mobile devices to conversational interactions on computers or mobile devices to conversational interactions that can be conducted via voice, text, or even images and gestures,’’ Etlinger says.
Although “many of the current uses cases are still primitive,” she believes, the shift is occurring with voice agents and chatbots today. And Etlinger warns against mistaking nascence for lack of potential, citing research by Gartner that by 2020, 30% of web browsing will be done without a screen.
See Related: The Biggest Digital Disruption Is An AI Skills Shortage
DBS Bank, for one, has rolled out DBS digibank, an entirely mobile bank in India, eliminating the need for paper, physical signatures and physical branches. All of its customer service and payments are done via a conversational AI platform. The assistant handles 82 percent of customer requests and inquiries and assists over 1.8 million customers with managing money, tracking expenses, analyzing spending and improving financial literacy, the Altimeter report notes.
To make this possible, a DBS team built a list of over 10,000 questions customers might possibly ask with the goal of understand a customer’s intent, according to the Altimeter report. If a customer uses words that indicate frustration, the content of the conversation is transferred by the agent to a live person to resolve.
As organizations plot their enterprise AI strategies, the Altimeter report makes the following recommendations:
• Use clean, high-quality data
• Select clearly defined uses cases that are “rich in data” and have enough characteristics and patterns so that insight is possible
• Set clear priorities for talent and the tools used since two well-documented challenges with AI are immature tool sets and a scarcity of talent
• Take the view that AI will augment, rather than replace employees and rethink the capabilities and skills you will need in the future, and allocate humans and technologies accordingly
• Recognize that there are ethical implications of using AI, since machine learning algorithms can and have ingested bias.
This article was originally published in Enterprise Mobility Exchange.